This Week In AI: (February 3rd - 9th 2025)
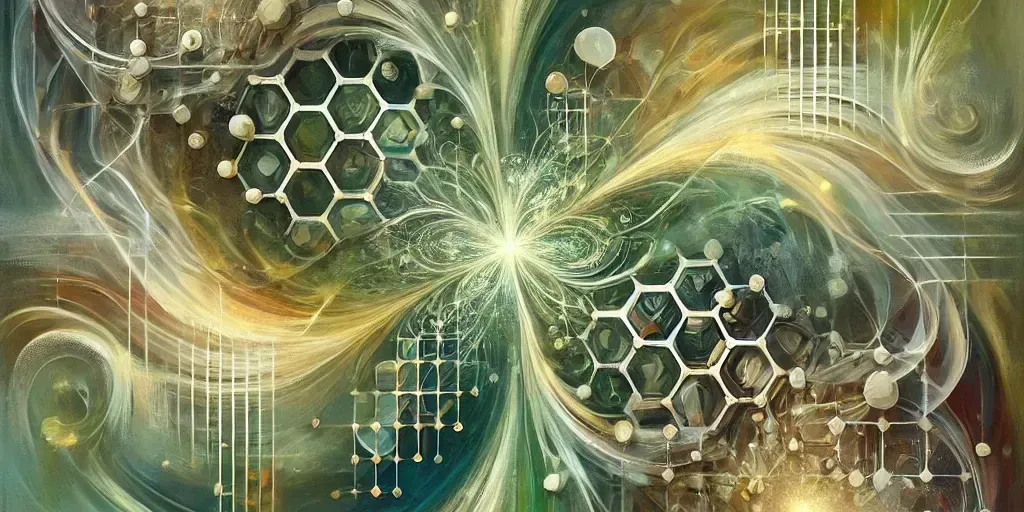
Table of Contents
This week in AI has been relatively quiet compared to previous weeks but we still saw another model release from OpenAI designed to do complex web-based research tasks, and Yann LeCun stated that he believes LLMs as we know them will be redundant in a few years. In research, a new interpretable loss function aims to replace the classic cross-entropy loss we all know and love, and researchers from HuggingFace advocate that fully autonomous AI agents should not be developed as they pose significant risks to humans and society.
OpenAI's Deep Research
After seeing multiple model launches last week by OpenAI, they released another this week- Deep Research! As you can see in the picture below, Deep Research is a new agentic style model that can perform complex, multi-step web-based research tasks. It is powered by a version of the new o3 reasoning model but specifically fine-tuned for web browsing tasks and data analysis.

The aim is for this model to be utilised heavily by professionals in fields such as finance, policy, etc. Since it can compile detailed reports on a wide range of topics, it will be perfect for people in these fields to automate certain tasks since it is the first OpenAI model that can provide clear citations (with fewer hallucinations). Deep Research has also now scored the highest accuracy on Humanity's Last Exam with 26.6%, only followed by o3-high. If you want a breakdown of Humanity's Last Exam, you can find it below. Simply, it is a new complex LLM benchmark.

Yann LeCun Think LLMs Will Be Redundant Soon
Meta's chief scientist and creator of the convolutional neural network, Yann LeCun, has made a bold claim that a "new paradigm of AI architectures" will emerge in the next three to five years. If you have listened to any talks by LeCun at any major AI conference (e.g., AAAI '24), you know he is a big doubter in current generative AI and large language model combinations.
He stated that "the shelf life of the current [LLM] paradigm is fairly short, probably three to five years", and he might not be wrong. We know that most open-source LLMs have drained the human corpus dry of information, once you have been trained on a large portion of the internet, what is left? There is growing talk in the field by researchers that LLMs are slowly reaching their ceiling, and it seems LeCun is a big believer in that!
Harmonic Loss for Interpretable AI
A new paper from MIT introduces harmonic loss, an alternative to the classic cross-entropy loss function used in training neural networks. Cross-entropy loss relies on inner product-based similarity metrics, but harmonic loss utilizes Euclidean distance, leading to scale invariance and a finite convergence point that can be interpreted as a class centre. These properties contribute to faster convergence and enhanced interpretability of the models. Just check out some of the weights when the network is passed test images from the MNIST dataset.

A network trained on harmonic loss has faster convergence, reduced grokking, and improved embeddings and weight interpretability to networks trained on cross-entropy loss. I did a deep dive in this week's Paper Spotlight, so if you want more information, check it out below!

Fully Autonomous AI Agents Should Not be Developed
A new paper from researchers out of Huggingface argues that AI systems with complete autonomy pose significant risks that outweigh their potential benefits. The study provides an analysis of AI autonomy, concluding that the more control users cede to AI agents, the greater the risks. Among the most concerning issues highlighted are safety risks (loss of human life), security vulnerabilities (hijacking), privacy violations, and the erosion of human oversight.
The paper outlines a four-tier framework for AI agent autonomy, ranging from simple rule-based assistants to fully autonomous systems capable of writing and executing their own code without human intervention. While semi-autonomous AI (such as task assistants) offers a favourable balance of benefits and risks, the authors warn that fully autonomous AI agents could lead to cascading failures, misinformation, and even dangerous system takeovers. The study also references historical cases where automation failures nearly led to catastrophic outcomes, reinforcing the argument for keeping human oversight in critical AI applications.
The authors advocate for technical and policy safeguards, emphasizing the need for clear limitations on AI autonomy. They argue that AI development should prioritize transparency, human control, and safety verification mechanisms to prevent unintended consequences. This paper adds to the growing debate on AI governance, calling for responsible development that aligns with ethical and societal values.