This Week In AI: (February 17th - 23rd 2025)
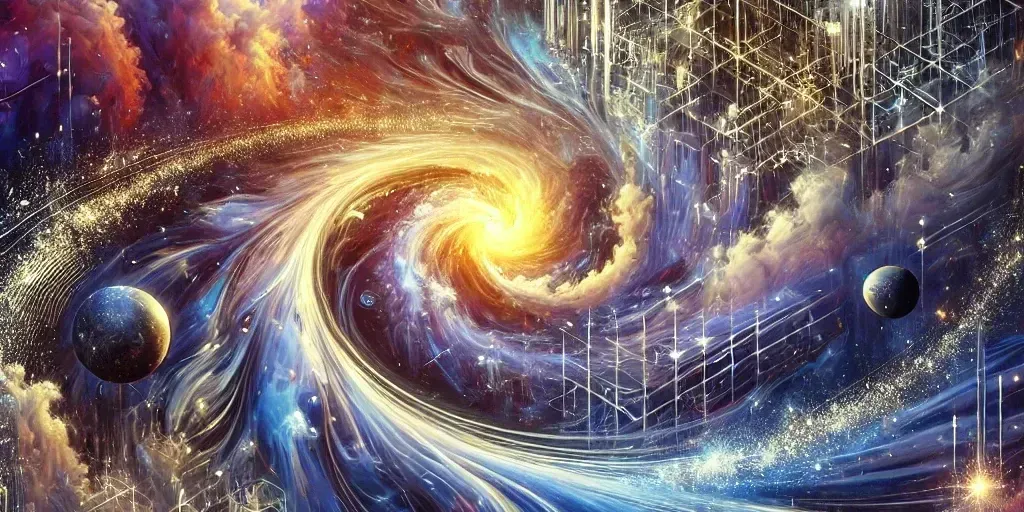
Table of Contents
This week in AI, we saw the unveiling of Grok 3, OpenAI hit another milestone, the end of AI wearables, and a brand new quantum chip from Microsoft which could change technology for decades. In research, LLMs were found to output small fingerprints when prompted, noise conditioning might not be needed in generative models, and your brain activity can now be converted into text!
Elon Musk's xAI Unveils Grok 3
Elon Musk's artificial intelligence startup, xAI, has launched its latest AI model, Grok 3, aiming to set new standards in the competitive AI chatbot landscape. Grok 3 is designed to rival leading models from OpenAI, DeepSeek, and Google, offering advanced text generation, image creation, and complex reasoning capabilities.
Grok 3 introduces several innovative modes to cater to diverse user needs. Think Mode activates Grok 3's reasoning process, allowing it to deconstruct complex problems into smaller, manageable steps. This mode is ideal for tasks requiring detailed analysis and step-by-step solutions. Big Brain Mode utilizes the full computational power of Grok 3 for intricate queries, providing more accurate and insightful responses. This mode is handy for scientific research and multifaceted problem-solving scenarios. DeepSearch enables Grok 3 to access real-time internet information, enhancing its responses with up-to-date data. This feature is beneficial for tasks like news aggregation, market analysis, and fact-checking.
In early evaluations, Grok 3 has demonstrated superior performance, reportedly surpassing OpenAI's GPT-4o and DeepSeek's V3 in areas such as mathematics, science, and coding. The model was trained using ten times more computing power than its predecessor, Grok 2, leveraging xAI's extensive data centre infrastructure.

OpenAI Reaches 400m Weekly Users
OpenAI's ChatGPT has reached a significant milestone, now serving over 400 million weekly active users as of this week. This marks a 33% increase from the 300 million users reported in December 2024. The AI chatbot market is becoming increasingly competitive, with new entrants like China's DeepSeek introducing models that claim to rival existing technologies at a fraction of the cost. Despite this, OpenAI continues to experience robust growth.
Microsoft's Majorana 1 Chip
Microsoft has unveiled the Majorana 1 chip, a groundbreaking advancement in quantum computing that leverages a novel state of matter known as a topological superconductor. This innovation aims to enhance the stability and scalability of qubits, the fundamental units of quantum information, potentially accelerating the development of practical quantum computers.

The integration of quantum computing with artificial intelligence has always held transformative potential across various sectors. Maybe this is a step closer towards the realization of large-scale, fault-tolerant quantum computers. Its successful integration could revolutionize AI by providing the computational power necessary to tackle problems currently beyond the reach of classical computers.
Humane's AI Pin Gets Bricked
Humane Inc. has announced that its AI Pin devices will cease functioning on February 28, 2025, following the company's acquisition by HP for $116 million. This shutdown will render features such as calls, messaging, and AI queries inoperable, effectively "bricking" the devices. Refunds are available only for purchases made within 90 days of the shutdown announcement, leaving many early adopters without compensation.
The demise of the Humane AI Pin is just the latest in a long line of AI-powered wearables that have failed to gain mainstream adoption. Devices like the Amazon Echo Loop, Google Glass, and Meta’s Ray-Ban Smart Glasses have all struggled due to a mix of high costs, limited functionality, and unclear consumer demand. Many of these products promise hands-free AI interactions, yet they often fail to offer a compelling use case that outperforms smartphones. Privacy concerns, short battery life, and reliance on cloud-based services further complicate adoption. With the AI Pin’s failure, it’s clear that companies entering the AI wearable space must address these fundamental challenges if they hope to succeed.
Brain-to-Text Decoding
A new paper, "Brain-to-Text Decoding: A Non-invasive Approach via Typing" introduces Brain2Qwerty, an AI-driven system capable of converting brain activity into text with unprecedented accuracy using non-invasive methods. Developed by researchers at Meta AI, the system leverages electroencephalography (EEG) and magnetoencephalography (MEG) recordings while participants type sentences on a keyboard. Unlike previous invasive brain-computer interfaces (BCIs), this method avoids the risks of neurosurgery while significantly improving decoding accuracy.
The study tested 35 healthy volunteers, measuring their brain activity as they typed memorized sentences. Brain2Qwerty, a three-stage deep learning model, was trained to decode these signals into text. Results showed MEG-based decoding achieved a character-error-rate (CER) of 32%, significantly outperforming EEG-based decoding, which had a 67% CER. The best-performing participants using MEG reached a CER as low as 19%, enabling near-perfect sentence reconstruction.
The research narrows the gap between invasive and non-invasive BCIs, offering a scalable, safer alternative for patients with neurodegenerative diseases, paralysis, or locked-in syndrome. While invasive BCIs achieve higher accuracy and real-time decoding, Brain2Qwerty demonstrates that non-invasive systems, particularly with MEG, can provide meaningful language decoding. Future work aims to adapt the system for real-time applications and clinical use. If successful, this technology could revolutionize assistive communication for individuals who have lost their ability to speak or type.
Idiosyncrasies in Large Language Models
A paper from Carnegie Mellon University explores a surprising phenomenon in AI: large language models (LLMs) such as ChatGPT, Claude, Grok, Gemini, and DeepSeek generate text in uniquely identifiable ways. By fine-tuning a text classifier on AI-generated content, researchers found that they could accurately determine which model produced a given text with 97.1% accuracy. This finding suggests that each AI model develops distinct linguistic patterns—akin to a "fingerprint"—that persist even when texts are rewritten, summarized, or translated.
The study attributes these idiosyncrasies to word choice, sentence structure, formatting tendencies, and even semantic preferences embedded in LLMs during their training. For example, ChatGPT tends to produce detailed explanations with structured markdown formatting, while Claude often prioritizes conciseness and clarity. These stylistic differences persist even after significant transformations, indicating that they are not merely surface-level artefacts but deeply embedded in the model’s learned representations. This research has far-reaching implications for AI safety, model evaluation, and synthetic data training. If AI-generated text carries inherent signatures, then training new models on synthetic data may reinforce these biases, potentially limiting model diversity or even making models more vulnerable to detection and tracking.
Is Noise Conditioning Necessary for Denoising Generative Models?
For years, denoising diffusion models—the backbone of many AI-generated images—have relied on noise conditioning to function properly. This means that during training, the model is explicitly told how much noise is in an image, helping it learn how to clean it up at different stages. But a new paper out of MIT, "Is Noise Conditioning Necessary for Denoising Generative Models?", challenges this long-standing assumption. The researchers found that many diffusion models still work surprisingly well even when you remove noise conditioning altogether—and some even perform better without it.
The paper tested multiple generative models, comparing versions that were given noise-level information to versions that weren’t. The expectation was that removing noise conditioning would cause major performance drops. Instead, the results showed only minor quality degradation in most models, and in some cases, flow-based methods actually improved. This raises an important question: Is noise conditioning really essential, or is it just something we’ve been doing because it’s always been done?

This work could open the door for simpler, more efficient generative models that produce high-quality images without needing explicit noise-level information. If AI models can generate just as well—or better—without noise conditioning, it might be time to rethink how we design diffusion models from the ground up.