Elon Musk claims we have exhausted AI training data
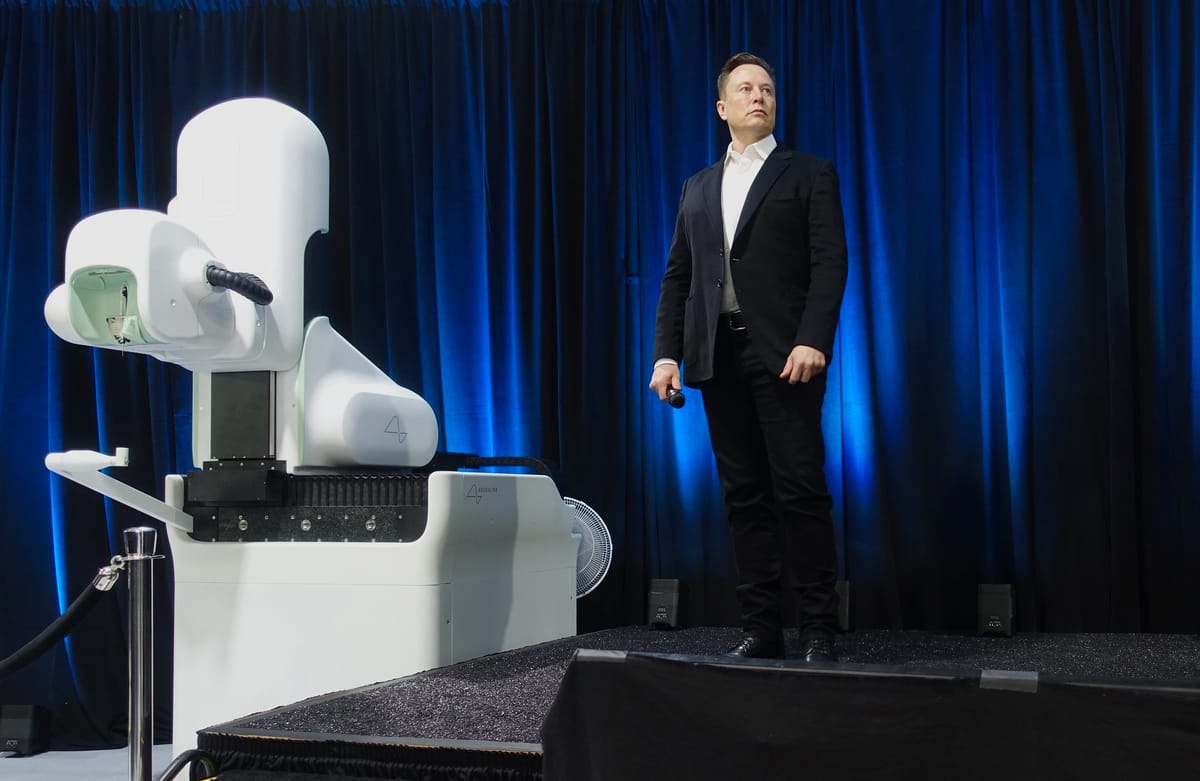
Elon Musk, the entrepreneur behind Tesla, and SpaceX made a bold claim in a recent interview: "We’ve now exhausted, all of the, basically, the cumulative sum of human knowledge has been exhausted in AI training” (stated in a Las Vega CES interview conducted by Mark Penn, posted online on X, January 8, 2025). We have heard the same argument made in the journal Nature recently where Nicola Jones stated "The Internet is a vast ocean of human knowledge, but it isn’t infinite. And artificial intelligence (AI) researchers have nearly sucked it dry”. Could this be true? Is the AI wave over? We think no, and let's dive into why!
Over the past few years, we have seen a major explosive growth of powerful AI systems being available for public use. We only need to look at large language models (LLMs) like OpenAI's ChatGPT models, or video and image generative models like SORA and DALL.E for superhuman examples. According to Musk, this growth has rapidly consumed the corpus of human knowledge available online and there is very little left to create the next generation of models.
For those who are unfamiliar with LLMs and how they are trained, LLMS are a category of AI that are trained to understand and generate human-like text. They are typically trained and fine-tuned on subsets of the internet and other massive datasets comprising various books, articles, and other sources of media. This is typically why you have heard models like ChatGPT do not know what they are saying, they are only regurgitating what they have read on the internet. You've heard this because it is more than often true. When given a prompt, the LLM predicts the most likely sequence of words to follow and that sequence is typically found in the training data. This is also the reason why students who blindly copy ChatGPT and other LLMs are often in trouble for plagiarism because the LLM itself is plagiarising its training data. Their reliance on vast quantities of training data makes them vulnerable to the issue Musk and other experts are highlighting: once the model has been trained on most of the internet, what is left?
Training LLMs also brings up another worrying issue: and that is LLMs being trained on a feedback loop. I am sure we have all seen the "dead internet theory", which states that a large portion of internet usage (posts, comments, etc) is being written by bots/AI models. Whilst this is definitely true, the extent to how bad it is is what is up for debate. The issue here is that if new iterations of LLMs are being trained on subsets of the internet and the internet is slowly becoming more populated by AI-generated content, the LLMs will be slowly trained on a feedback loop from themselves. The phenomenon is known as "model cannibalization", and feedback loops in model training are a very large issue as over time the data degrades in quality. Eventually if left unattended, ChatGPT could potentially go from saying logical sentences to random letters.
Given these issues of little training data left and model cannibalization, we have to look towards new ideas to improve future iterations of AI systems. AI experts suggest we should look to other training techniques other than raw data. Some future directions could be:
- Raw interaction: Currently LLMs learn from other people's interactions with each other on the internet and use that as some of its training data. Even with its flaws, we have seen techniques such as reinforcement and imitation learning provide superhuman abilities through interaction alone. Just look at AlphaGo and AlphaFold as proof. If the LLM had the ability to learn from its current training corpus but also could interact with an environment like a pseudo version of the internet, it could further improve itself and generate its own synthetic training data.
- Reasoning and logic: With developments like the ChatGPT o1 model, it is clear that improving the model's ability to reason and deduce its own logic is a powerful ability. Moving beyond simple pattern recognition to develop the model's own understanding of concepts could be the future.
- Novel solutions: Examples like AlphaGo's move 37 show that letting an AI system learn its own optimal behaviour and concepts leads to solutions more powerful than our human understanding. When we add human knowledge to the training of the model, there is the potential for the model to get stuck in a local optima and not surpass human abilities and we have essentially constrained it with our subpar human knowledge. Future research could look into using less and less training data from humans (such as the internet) and allow the model to initialize itself from the environment for the potential to surpass our feasible human knowledge instead of regurgitating it.
Elon Musk’s assertion that AI has exhausted its training data is a stark reminder of the challenges facing the field. As the AI community grapples with this reality, it must innovate and adapt to avoid stagnation. Whether through the digitization of new knowledge, enhanced human-AI collaboration, or regulatory oversight, the solutions will require unprecedented cooperation across industries and borders.
- Musk and AI experts have claimed we have exhausted all of the human knowledge training data to train AI models.
- The future could see models begin to train on a feedback loop from themselves as AI content becomes prevalent on the internet.
- Future research should look towards improving reasoning and allowing the model to directly interact with an environment to surpass human abilities.